The goal of any incentive program is to create net positive value for the protocol. In the case of Automated Market Makers (AMMs), this usually means generating a sustained boost in liquidity and market share. Since an AMM accrues value proportional to its usage by Liquidity Providers (LPs) and traders, a long-term increase in usage is most likely to generate a net positive return over time.
Historically, it is a tough challenge for AMMs to retain the growth created through incentives for the long term. Achieving rapid growth by spending indiscriminately is fairly straightforward, but these gains often disappear as soon as the incentive program ends. Historically, many AMM incentive programs have only had a temporary effect, but this does not necessarily have to be the case.
Gauntlet approaches AMM incentives by first understanding where growth can become self-sustaining. We can then use incentives in a targeted way to most effectively pursue this end goal.
Types of Incentive Programs
There are broadly two ways to go about AMM incentives, depending on which participants in the ecosystem are being rewarded. Trade Mining programs attract traders by rewarding them for trading with the AMM, while Liquidity Mining programs attract LPs by rewarding them for the liquidity they supply.
Some designs may combine elements of Trade Mining and Liquidity Mining, but for simplicity, we can consider the two mechanisms separately. The long-term impact of incentives depends on how “sticky” traders or LPs are after the programs have concluded. By understanding what makes trader or LP usage self-sustaining, we can then decide what type of incentive program is most likely to achieve the desired impact.

Trade Mining Programs
In the domain of Trade Mining programs, some AMMs have experimented with directly paying traders for volume. The goal of this would be to onboard new traders who would then continue using the platform after the reward period has ended. The main challenges to this type of program are:
- User Elasticity – Most traders seek out the best execution possible for their trades. Generally, it is unlikely that traders will continue using a specific AMM if there are cheaper options elsewhere. Unless the AMM can provide superior execution without incentives, this makes the stickiness of traders doubtful beyond the end of a Trade Mining program.
- Lack of Sybil Resistance – If the rewards are of material value, they likely exceed the expected cost of making a random trade, incentivizing users to inorganically boost their trading volume. Unless wash trades and adversarial users can be identified and filtered from the rewards distribution, this is very inefficient for the program overall.
Our recent analysis of trading on decentralized exchanges (DEXs) found that the vast majority of traders are simply driven by execution quality. In this environment, trade mining programs do not make much sense because there is little stickiness from traders and any growth would likely be temporary.
Further, Trade Mining programs are exploitable by users and hard to design efficiently. In theory, rewards could be limited to a small percentage of trade volume so as to reduce friction but not incentivize inorganic trading. In practice, however, it is difficult to prevent some degree of reward farming that brings no long-term value to the protocol. Due to these challenges, Trade Mining has not seen much use in AMM incentive design outside of a few rare examples.
Liquidity Mining Programs
Currently, Liquidity Mining is popular across DeFi as the dominant approach for driving AMM growth. This usually involves rewarding LPs based on the amount of liquidity they provide and the time or usage their liquidity attains in the protocol. Though the historical track record of Liquidity Mining is mixed, there has not been much quantitative planning behind these programs in the past. With better preparation and analysis, Liquidity Mining could be a more efficient tool than it has been so far. In designing Liquidity Mining programs, there are two mechanisms we have identified that can create long-term sustained impact:
- Forgetful LPs – users who “forget” to withdraw their liquidity after the incentive program ends or continue providing liquidity for any reason unrelated to expected return
- Liquidity Bootstrapping – a self-sustaining cycle where LPs attract organic trading, which creates a track record of strong volume and LP returns for the pool, which then continues to attract LPs once the incentive period ends.
While most previous research on Liquidity Mining has focused on forgetful LPs, Gauntlet sees liquidity bootstrapping as the more promising mechanism. We have observed that LPs do seem to be sticky in some cases, and theorize that liquidity bootstrapping is the main driver behind this.

Our main divergence from past analysis lies in measuring pool performance relative to competing pools, as opposed to using an absolute metric. Since natural variation in the overall market is a confounding factor in assessing growth, we view the normalized TVL and volume relative to similar pools as a more accurate objective.
Our recent analysis of historical Liquidity Mining on Uniswap found that when benchmarked appropriately, the effort was more successful than initially believed. Though pools were selected essentially at random, 2 of the 5 pools we studied did show positive signs of liquidity bootstrapping. By targeting incentives with simulation modeling, we aim to increase the impact and success rate of Liquidity Mining programs. Due to the self-sustaining nature of liquidity gains, Gauntlet sees this as the more promising way to design programs that generate net positive returns to the protocol over time.
Blog
View the full presentation
Read the full paper
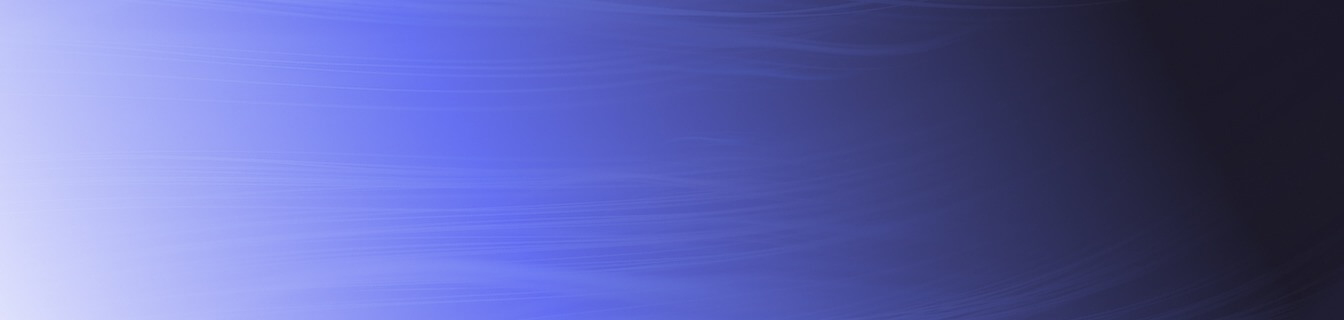